视频选集
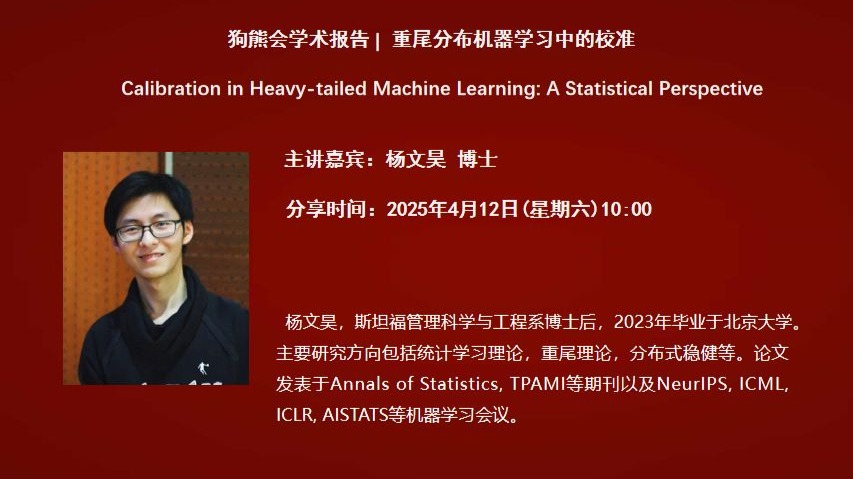
Heavy-tailed noise has gained significant attention in modern machine learning due to its impact on generalization performance in stochastic training algorithms, especially stochastic gradient descent (SGD). While empirical evidence suggests that heavy-tailed noise can enhance optimization dynamics and improve model generalization, it also poses fundamental challenges for theoretical analysis and statistical inference.
In this talk, I will present a statistical perspective on the role of heavy-tailed noise in SGD. Specifically, I will discuss the asymptotic properties of SGD under heavy-tailed noise, highlighting key differences from classical light-tailed theory. These results provide new insights into the calibration of learning algorithms under non-standard conditions, bridging the gap between optimization and statistical inference. I will conclude by discussing the implications of these findings for practical machine learning applications, highlighting open questions and future research directions in the intersection of optimization, statistical inference, and robustness in heavy-tailed settings. This talk is based on joint works with Jose Blanchet, Peter Glynn and Aleksandar Mijatović.
嘉宾简介
杨文昊,斯坦福管理科学与工程系博士后,2023年毕业于北京大学。主要研究方向包括统计学习理论,重尾理论,分布式稳健等。论文发表于Annals of Statistics, TPAMI等期刊以及NeurIPS, ICML, ICLR, AISTATS等机器学习会议。
直播分享时间:2025年4月12日